El cálculo vectorial, análisis vectorial o cálculo multivariable es un campo de las matemáticas referidas al análisis real multivariable de vectores en 2 o más dimensiones. Es un enfoque de la geometría diferencial como conjunto de fórmulas y técnicas para solucionar problemas muy útiles para la ingeniería y la física.
Consideramos los campos vectoriales, que asocian un vector a cada punto en el espacio, y campos escalares, que asocian un escalar a cada punto en el espacio. Por ejemplo, la temperatura de una piscina es un campo escalar: a cada punto asociamos un valor escalar de temperatura. El flujo del agua en la misma piscina es un campo vectorial: a cada punto asociamos un vector de velocidad.
Cuatro operaciones son importantes en el cálculo vectorial:
- Gradiente: mide la tasa y la dirección del cambio en un campo escalar; el gradiente de un campo escalar es un campo vectorial.
- Rotor o rotacional: mide la tendencia de un campo vectorial a rotar alrededor de un punto; el rotor de un campo vectorial es otro campo vectorial.
- Divergencia: mide la tendencia de un campo vectorial a originarse o converger hacia ciertos puntos; la divergencia de un campo vectorial es un campo escalar.
- Laplaciano: relaciona el "promedio" de una propiedad en un punto del espacio con otra magnitud, es un operador diferencial de segundo orden.
La mayoría de los resultados analíticos se entienden más fácilmente usando la maquinaria de la geometría diferencial, de la cual el cálculo vectorial forma un subconjunto.
Historia
El estudio de los vectores se origina con la invención de los cuaterniones de Hamilton, quien junto a otros los desarrollaron como herramienta matemáticas para la exploración del espacio físico. Pero los resultados fueron desilusionantes, porque vieron que los cuaterniones eran demasiado complicados para entenderlos con rapidez y aplicarlos fácilmente.
Los cuaterniones contenían una parte escalar y una parte vectorial, y las dificultades surgían cuando estas partes se manejaban al mismo tiempo. Los científicos se dieron cuenta de que muchos problemas se podían manejar considerando la parte vectorial por separado y así comenzó el Análisis Vectorial.
Este trabajo se debe principalmente al físico estadounidense Josiah Willard Gibbs (1839-1903) y al físico matemático inglés Oliver Heaviside(1850-1925).
Cálculo diferencial en campos escalares y vectoriales
Funciones de Rn en Rm. Campos escalares y vectoriales
Formularemos las definiciones para campos vectoriales. También serán válidas para campos escalares. Sea

un campo vectorial que hace corresponder a todo punto P definido biunívocamente por su vector posición, un vector
donde el punto O es nuestro origen de coordenadas.
con
y
. Cuando
tenemos un campo escalar. Para
tenemos un campo vectorial. Utilizaremos la norma euclídea para hallar la magnitud de los vectores.
Límites y continuidad
Si
y
Escribimos:
,
- o bien,
cuando 
- para expresar lo siguiente:
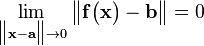
donde
es la norma euclídea de
. Expresándolo en función de las componentes de 

o, de forma equivalente,
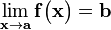
Decimos que una función es continua en 
|
Demostración |
Sabemos que a) y b) en el teorema se verifican si y son funciones escalares. Por tanto, si
tenemos
![\begin{array}{rl}
a) & \mathbf{f}\big (\mathbf{x}) = \big [f_1\big (\mathbf{x}\big ),\ldots,f_m\big (\mathbf{x}\big )\big ],
\mathbf{g}\big (\mathbf{x}) = \Big [g_1\big (\mathbf{x}\big ),\ldots,g_m\big (\mathbf{x}\big )\Big ] \\
& \lim_{\mathbf{x} \to \mathbf{a}}\big (\mathbf{f} + \mathbf{g}\big )\big (\mathbf{x}\big ) =
\lim_{\mathbf{x} \to \mathbf{a}}\Big [\big (f_1+g_1\big )\big (\mathbf{x}\big ),\ldots,\big (f_m+g_m\big )\big (\mathbf{x}\big )\Big ] = \\
& \Big [\lim_{\mathbf{x} \to \mathbf{a}}\big (f_1+g_1\big )\big (\mathbf{x}\big ),\ldots,\lim_{\mathbf{x} \to \mathbf{a}}\big (f_m+g_m\big )\big (\mathbf{x}\big )\Big ] = \\
& \Big [\lim_{\mathbf{x} \to \mathbf{a}}f_1\big (\mathbf{x}\big )+ \lim_{\mathbf{x} \to \mathbf{a}}g_1(\mathbf{x}\big ),\ldots,\lim_{\mathbf{x} \to \mathbf{a}}f_m\big (\mathbf{x}\big ) + \lim_{\mathbf{x} \to \mathbf{a}}g_m\big (\mathbf{x}\big )\Big ] = \\
& \big (b_1+c_1,\ldots,b_m+c_m\big ) = \big (b_1,\ldots,b_m\big )+\big (c_1,\ldots,c_m\big ) = \mathbf{b}+\mathbf{c}
\end{array}](/images/math/f/8/d/f8de70077b8bd85230e34905ac818219.png)
![\begin{array}{rl}
b) & \lim_{\mathbf{x} \to \mathbf{a}}\lambda\mathbf{f}\big (\mathbf{x}\big ) =
\lim_{\mathbf{x} \to \mathbf{a}}\lambda\Big [f_1\big (\mathbf{x}\big ),\ldots,f_m\big (\mathbf{x}\big )\Big ] =
\lim_{\mathbf{x} \to \mathbf{a}}\Big [\lambda f_1\big (\mathbf{x}\big ),\ldots,\lambda f_m\big (\mathbf{x}\big )\Big ] = \\
& \Big [\lim_{\mathbf{x} \to \mathbf{a}}\lambda f_1\big (\mathbf{x}\big ),\ldots,\lim_{\mathbf{x} \to \mathbf{a}}\lambda f_m\big (\mathbf{x}\big )\Big ] = \Big [\lambda\lim_{\mathbf{x} \to \mathbf{a}}f_1\big (\mathbf{x}\big ),\ldots,\lambda\lim_{\mathbf{x} \to \mathbf{a}}f_m\big (\mathbf{x}\big )\Big ] = \\
& \lambda\Big [\lim_{\mathbf{x} \to \mathbf{a}}f_1\big (\mathbf{x}\big ),\ldots,\lim_{\mathbf{x} \to \mathbf{a}}f_m\big (\mathbf{x}\big )\Big ] = \lambda\big (b_1,\ldots,b_m\big ) = \lambda\mathbf{b}
\end{array}](/images/math/0/5/2/052e65397a3035a6272d128544031afb.png)
![c) \quad \big (\mathbf{f}\cdot\mathbf{g}\big )\big (\mathbf{x}\big )-\mathbf{b}\cdot\mathbf{c} =
\Big [\mathbf{f}\big (\mathbf{x}\big )-\mathbf{b}\Big ]\cdot\Big [\mathbf{g}\big (\mathbf{x}\big )-\mathbf{c}\Big ]+
\mathbf{b}\cdot\Big [\mathbf{g}\big (\mathbf{x}\big )-\mathbf{c}\Big ]+\mathbf{c}\cdot\Big [\mathbf{f}\big (\mathbf{x}\big ) -\mathbf{b}\Big ]](/images/math/5/8/9/589371a0f58ec18d46ec76c6d91226d2.png)
- Aplicando la desigualdad triangular y la desigualdad de Cauchy-Schwarz tenemos

- , como queríamos demostrar.
, como queríamos demostrar.
|
Demostración |
Sean y . Entonces,
![\begin{array}{l}
\lim_{\big \|\mathbf{x}-\mathbf{a}\big \| \to 0}\Big \|\mathbf{f}\Big [\mathbf{g}\big (\mathbf{x}\big )\Big ]-\mathbf{f}\Big [\mathbf{g}\big (\mathbf{a}\big )\Big ]\Big \| = \lim_{\big \|\mathbf{y}-\mathbf{b}\big \| \to 0}\Big \|\mathbf{f}\big (\mathbf{y}\big )-\mathbf{f}\big (\mathbf{b}\big )\Big \|=0 \Rightarrow \\
\lim_{\mathbf{x} \to \mathbf{a}}\mathbf{f}\Big [\mathbf{g}\big (\mathbf{x}\big )\Big ]=\mathbf{f}\Big [\mathbf{g}\big (\mathbf{a}\big )\Big ]
\end{array}](/images/math/b/8/b/b8b099b84086d195e37f5936e7b92191.png)
- como queríamos demostrar.
|
Derivadas direccionales
Derivada de un campo escalar respecto a un vector
Derivadas parciales
- Si derivamos la expresión anterior respecto a una segunda variable,
, tendremos
. En la práctica, calcularemos
derivando respecto a
y suponiendo
constante.
La diferencial
Definición de campo escalar diferenciable
- La anterior ecuación es la fórmula de Taylor de primer orden para
.
Teorema de unicidad de la diferencial
Demostración |
- como queríamos demostrar.
Expresando en función de sus componentes en la base

- como queríamos demostrar.
|
Regla de la cadena
Diferencial de un campo vectorial
Expresando
en función de sus componentes, tenemos ![\mathbf{f'}\big (\mathbf{x};\mathbf{y}\big ) = \Big [f'_1\big (\mathbf{x};\mathbf{y}\big ),\ldots,f'_m\big (\mathbf{x};\mathbf{y}\big )\Big ]](/images/math/1/d/9/1d976d3392ce06dc5b66ffb1e28a7818.png)
- Esta es la fórmula de Taylor de primer orden para
.
La matriz de
es su matriz jacobiana.
Diferenciabilidad implica continuidad
- Se deduce fácilmente de la fórmula de Taylor de primer orden ya vista.
Regla de la cadena para diferenciales de campos vectoriales
Condición suficiente para la igualdad de las derivadas parciales mixtas
ambas derivadas parciales existen y son continuas en .
|
Aplicaciones del cálculo diferencial
Cálculo de máximos, mínimos y puntos de ensilladura para campos escalares
Un campo escalar tiene un máximo en existe una n-bola 
|
Un campo escalar tiene un mínimo en existe una n-bola 
|
Un campo escalar tiene un punto de ensilladura 
.
|
Función con un punto de ensilladura
Para saber si es uno de los casos anteriores:
- Obtenemos

- Obtenemos la matriz hessiana de f. Sea esta
.
es definida positiva
tiene un mínimo local (mínimo relativo) en
.
es definida negativa
tiene un máximo local (máximo relativo) en
.
es indefinida
tiene un punto de ensilladura en
.
En lo anteriormente expuesto, hemos supuesto que
es continua 
Véase también
En inglés: Vector calculus Facts for Kids